How AI is being used to assist the Pharma industry
Artificial Intelligence (AI) has evolved in no time at all from a concept of potential into a cornerstone of innovation across industries.
In the pharmaceutical sector, AI's applications have transitioned from experimental to indispensable, transforming the way drugs are discovered, patients are treated, and healthcare is delivered.
The pharmaceutical industry, traditionally plagued by high costs and lengthy timelines, is now embracing AI to optimize processes, reduce inefficiencies, and improve outcomes.
Challenges in Drug Discovery
Cost
The cost of developing a new drug has been estimated at $2.6 billion, according to a 2016 study by the Tufts Center for the Study of Drug Development.
However, this figure has faced criticism for potentially overstating costs by including opportunity costs and relying on private data.
While adjusted for inflation, the number remains a point of debate in the industry.
Time
It can take up to 10-15 years to develop a new drug.
This timeline includes discovery, preclinical testing, and clinical trials, and is widely accepted as reflective of the complex drug development process.
Complexity
The human body is incredibly complex, and it is difficult to predict how a new drug will interact with it.
Researchers must carefully consider a drug's potential side effects and interactions with other medications, as well as its efficacy in treating the targeted condition.
Data Volume
The amount of data generated in drug discovery is growing exponentially.
This includes genomic, proteomic, and clinical trial data, which can be challenging to manage and analyze.
AI helps researchers sift through this vast information to identify promising drug candidates and optimize their development.
AI in Drug Discovery
The process of discovering new drugs has historically been resource-intensive, requiring billions of dollars and over a decade to bring a single drug to market.
AI is revolutionizing this process in several ways:
- Drug Target Identification: AI can analyze vast amounts of genomic and proteomic data to identify potential drug targets, determining which proteins or genes a drug should target to address specific diseases effectively.
- Accelerating Lead Identification: By analyzing vast datasets, AI can identify potential drug candidates faster than traditional methods. Algorithms can process millions of chemical compounds to determine those most likely to be effective against a target disease.
- Lead Compound Optimization: AI helps optimize the structure of lead compounds by analyzing structure-activity relationships and suggesting modifications to enhance therapeutic properties, improving efficacy and safety.
- Predicting Drug Efficacy and Safety: Machine learning models analyze historical data to predict how a drug will perform in clinical trials, reducing the likelihood of late-stage failures.
- Clinical Trial Design: AI supports the design of more efficient clinical trials by optimizing patient selection, endpoint choices, and overall trial structures to maximize success rates and minimize costs.
- Patient Stratification: By analyzing patient data, AI can identify subgroups most likely to respond to a particular treatment, leading to more targeted and effective clinical trials. However, challenges such as limited data diversity can introduce biases, requiring careful consideration.
AI Beyond the Lab
AI's impact is not confined to the research and development phase; it is equally transformative in patient care and clinical operations.
- Patient Diagnostics and Treatment: AI-driven tools enable personalized medicine, tailoring treatment plans based on individual genetic profiles. Additionally, AI systems are advancing early disease detection, identifying subtle patterns that humans might overlook.
- Clinical Operations: AI automates routine tasks like data analysis and reporting, freeing up resources for higher-value activities. For example, AI-powered platforms enhance patient recruitment by identifying eligible participants more efficiently. Real-world evidence generation through AI tools also improves clinical decision-making.
Real-World Examples of AI in Pharma
Several companies are at the forefront of leveraging AI in pharma:
- Insilico Medicine: Specializes in using AI for drug discovery, recently identifying a novel drug candidate in record time.
- IBM Watson Health: While IBM has reduced its focus on Watson Health due to challenges, its earlier contributions to AI in healthcare remain notable.
- Exscientia: Credited with AI-designed drugs in clinical trials, such as a candidate reaching trials within months rather than years.
These implementations showcase AI's tangible impact, reducing time-to-market and improving patient outcomes.
However, providing specific achievements and dates can lend greater precision and clarity.
Challenges and Ethical Considerations
While AI offers immense promise, it also introduces new challenges:
- Data Privacy and Security: With AI relying on vast amounts of sensitive patient data, ensuring robust cybersecurity measures is paramount.
- Bias in Algorithms: AI systems can inherit biases from the data they are trained on, potentially leading to inequities in treatment. Addressing limited data diversity is critical to minimizing bias.
- Transparency and Explainability: The complexity of AI models can make it difficult to understand how decisions are made, raising concerns among regulators and practitioners.
Concrete examples, such as cases of biased treatment recommendations or data breaches, can illustrate these challenges more effectively.
Additionally, discussing the evolving regulatory landscape for AI-driven drug development can provide further insights.
The Future of AI in Pharma
The future of AI in the pharmaceutical industry is bright, with predictions pointing toward even greater integration into healthcare systems:
- Revolutionizing Drug Development: AI will likely play a role in every stage of the drug lifecycle, from discovery to post-market surveillance.
- Enhancing Patient Care: AI-driven tools will empower physicians with deeper insights, enabling more accurate diagnoses and personalized treatments.
- Fostering Collaboration: Partnerships between tech companies, pharmaceutical firms, and academic institutions will drive innovation and ensure that AI's potential is fully realized.
AI is a transformative force reshaping the pharmaceutical industry.
By addressing challenges such as data privacy and algorithmic bias, stakeholders can harness AI's power to accelerate drug discovery, enhance patient care, and improve clinical operations.
For industry leaders, the call to action is clear: embrace AI's potential, invest in its development, and foster a culture of collaboration to unlock the future of healthcare.
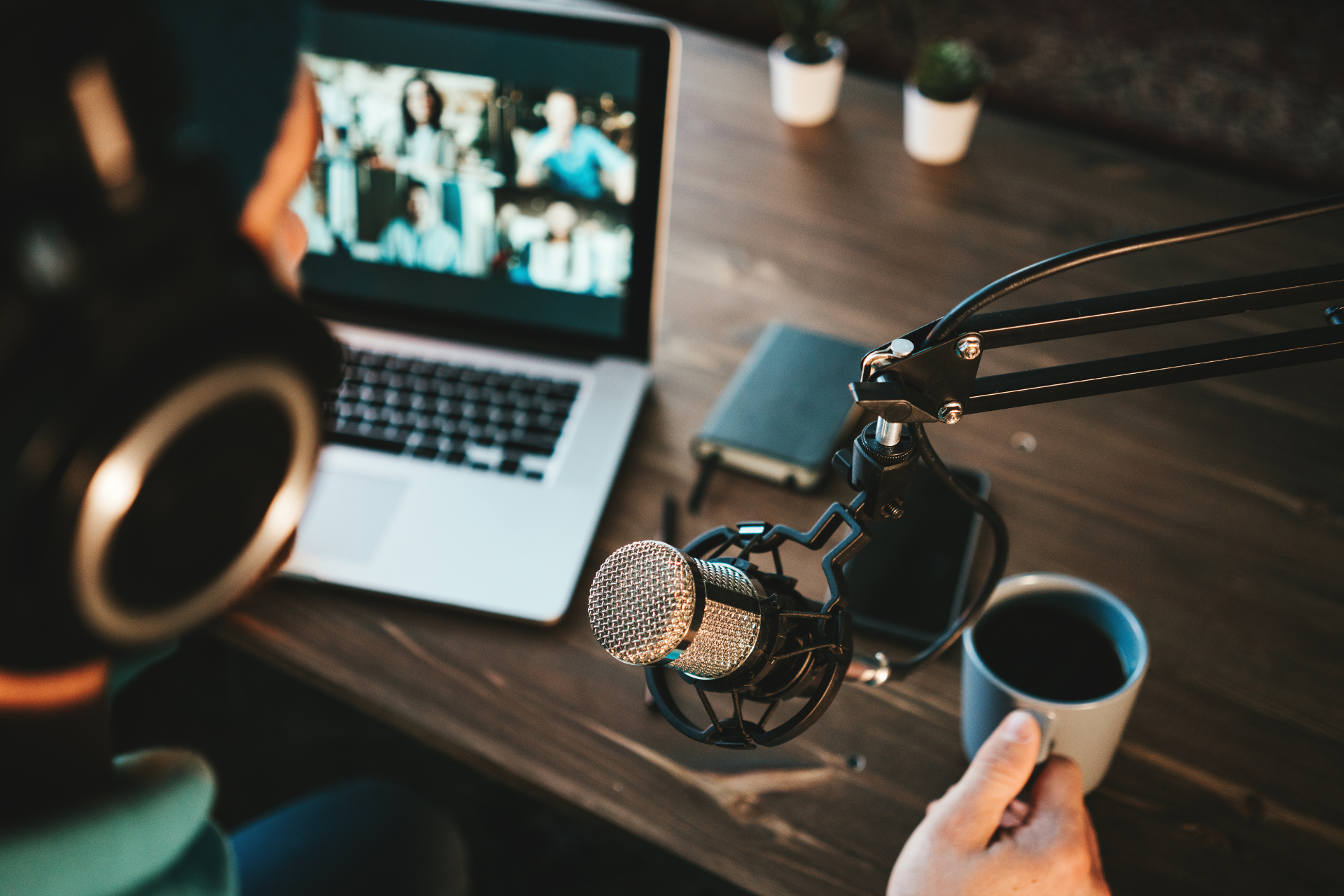
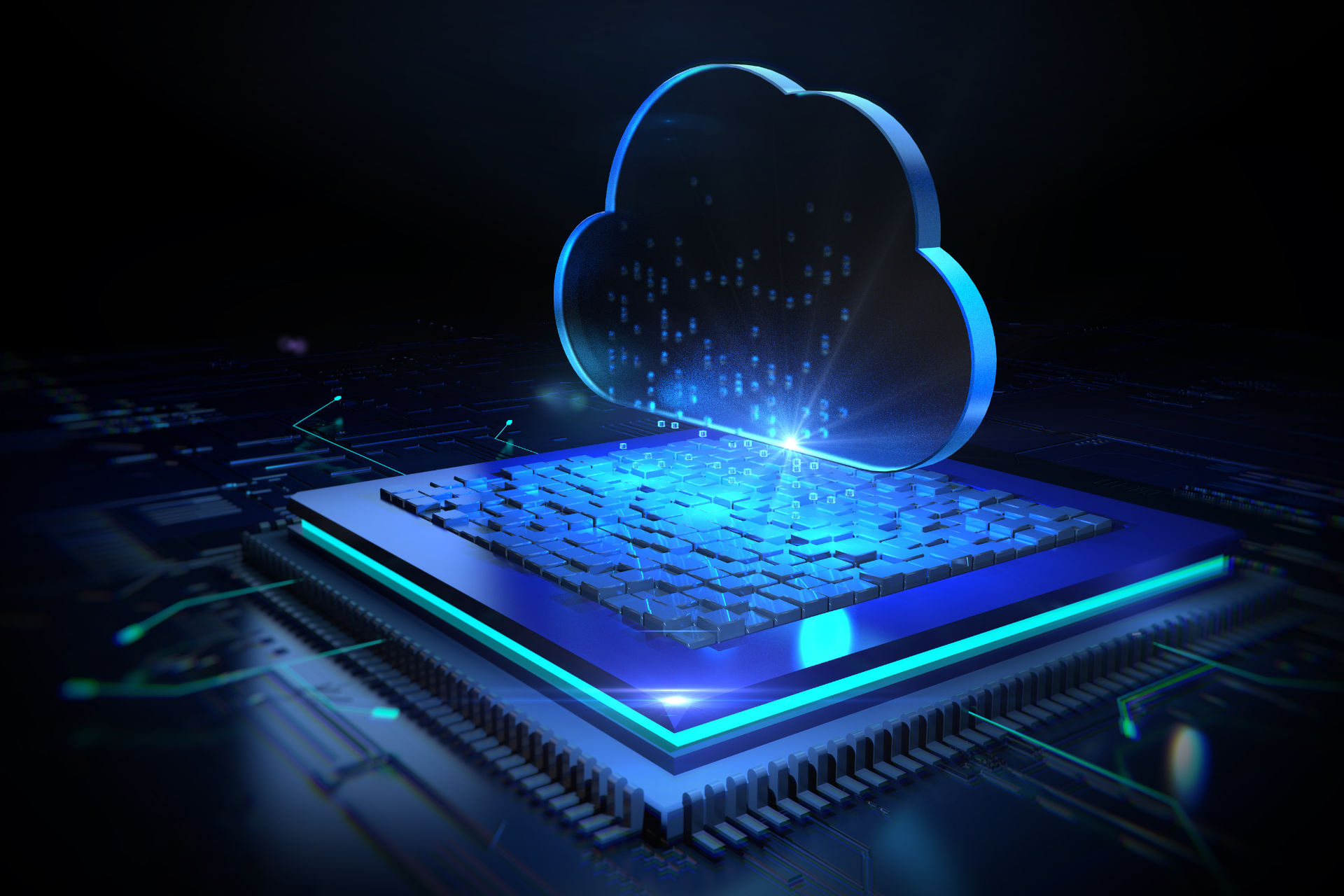
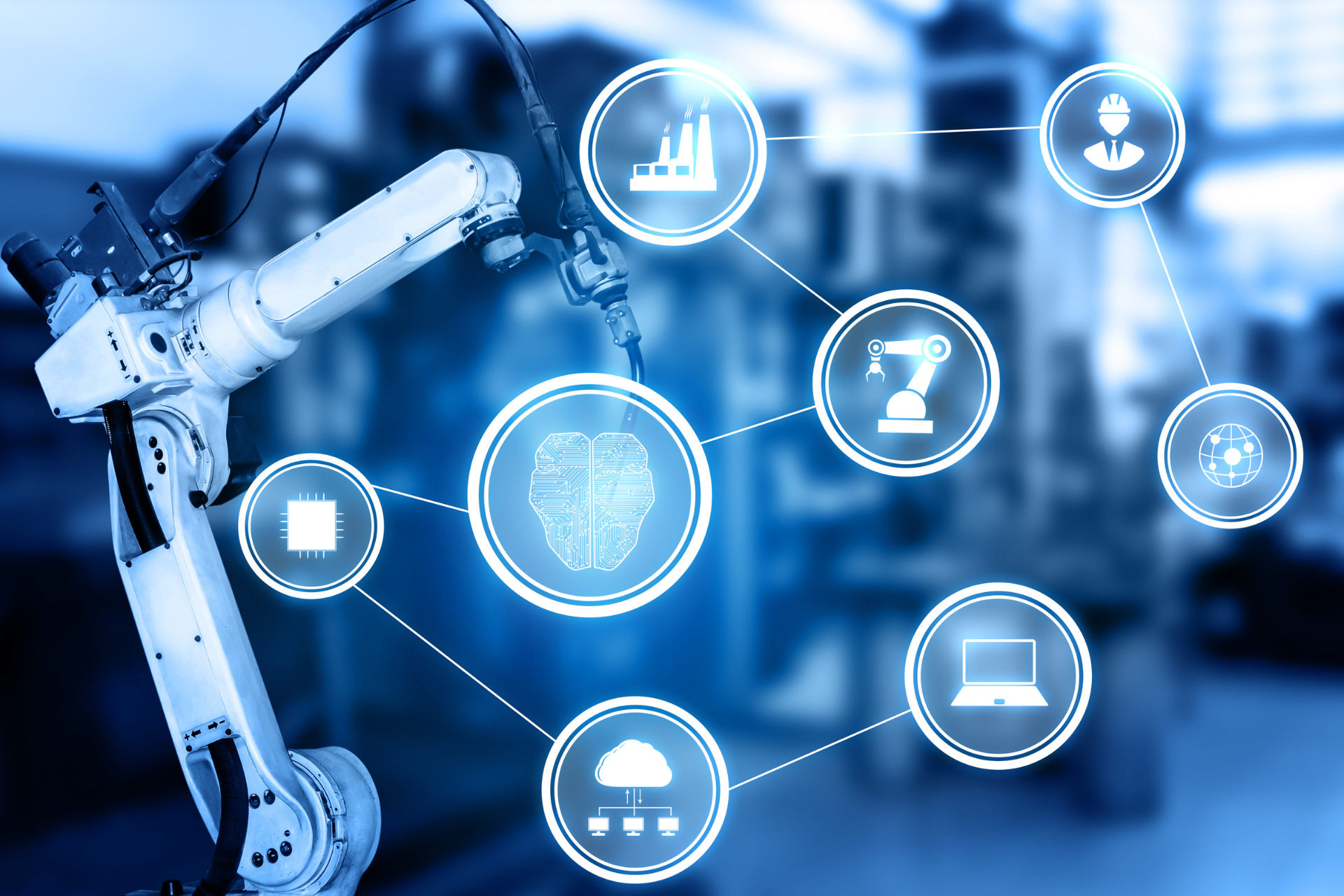
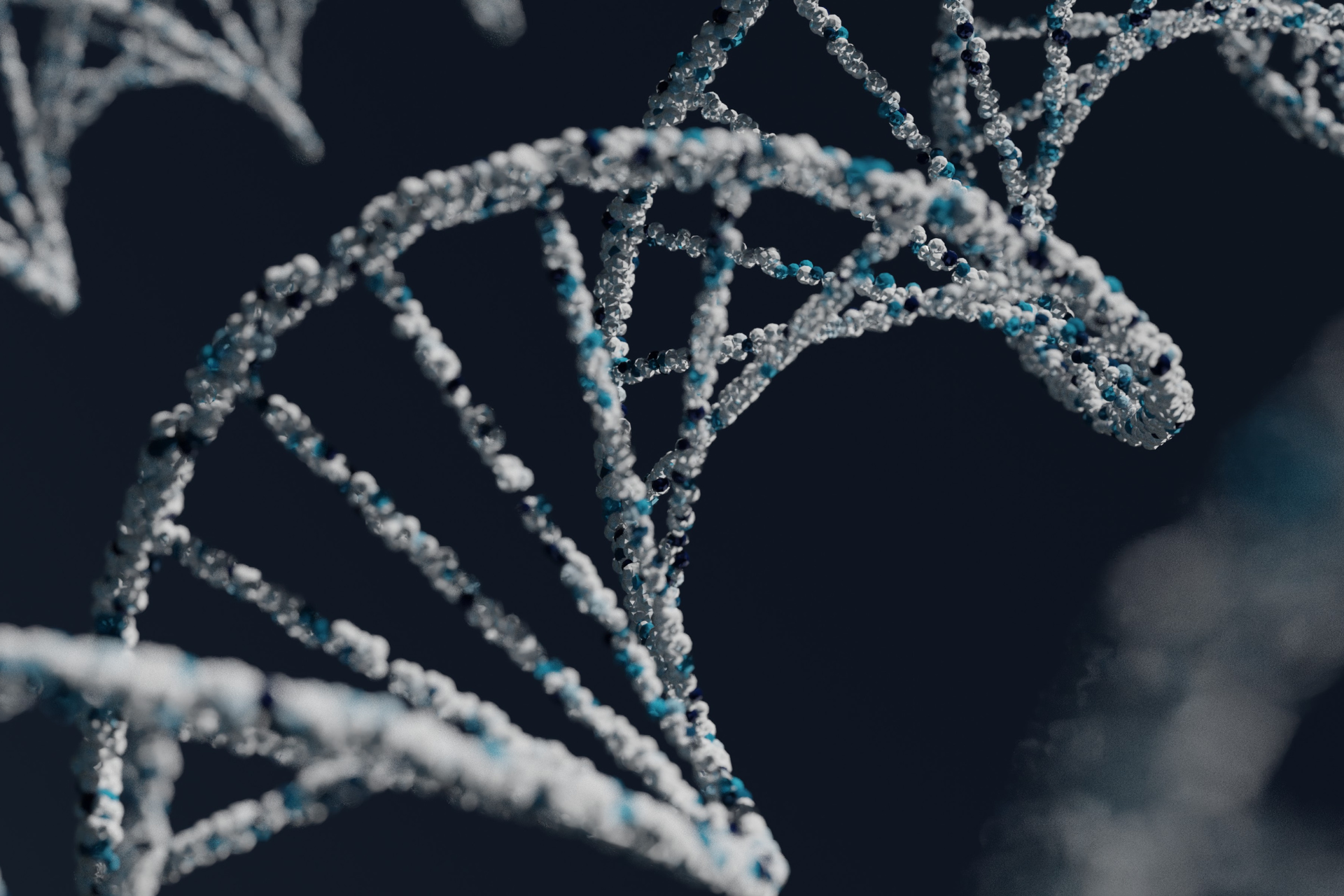
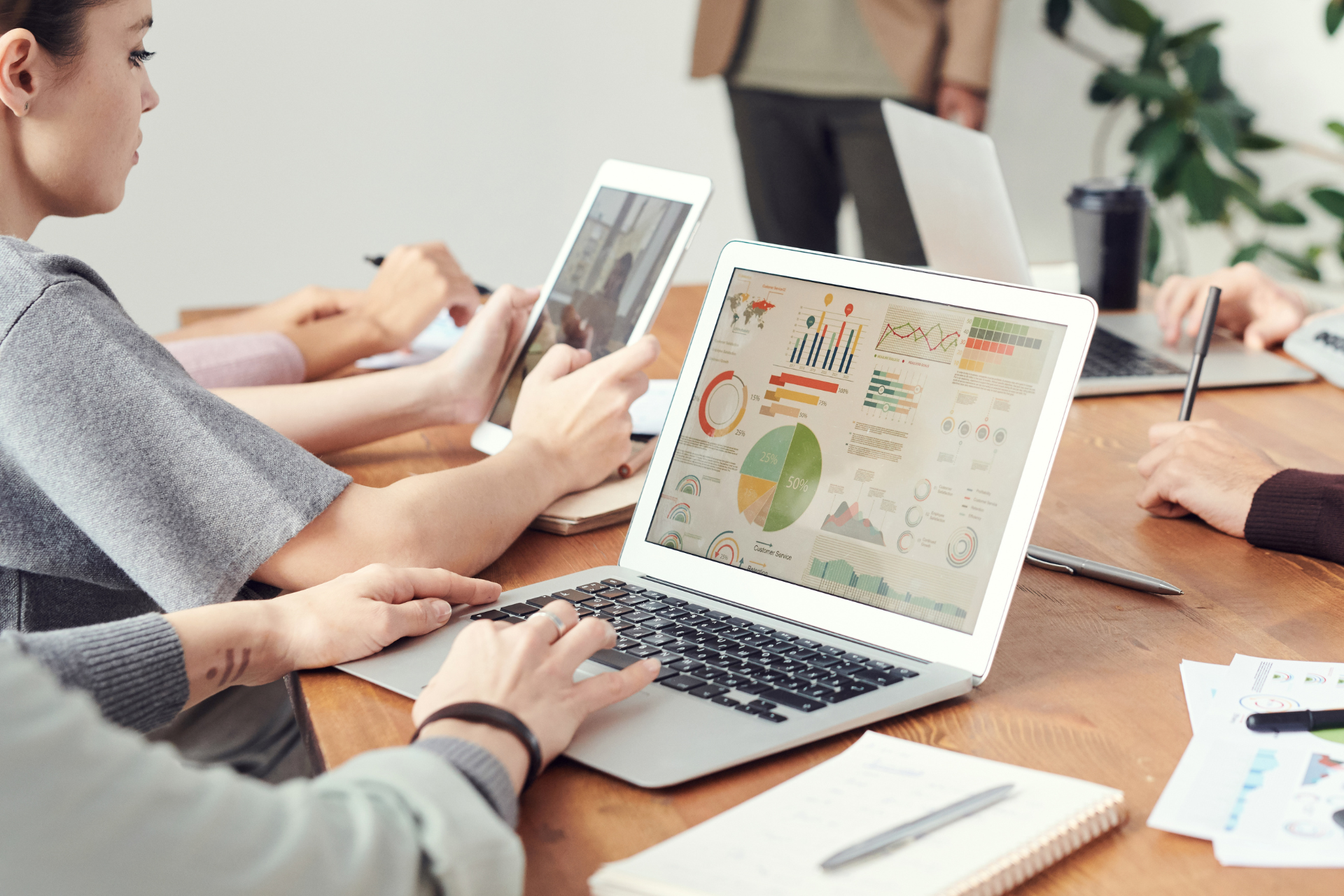
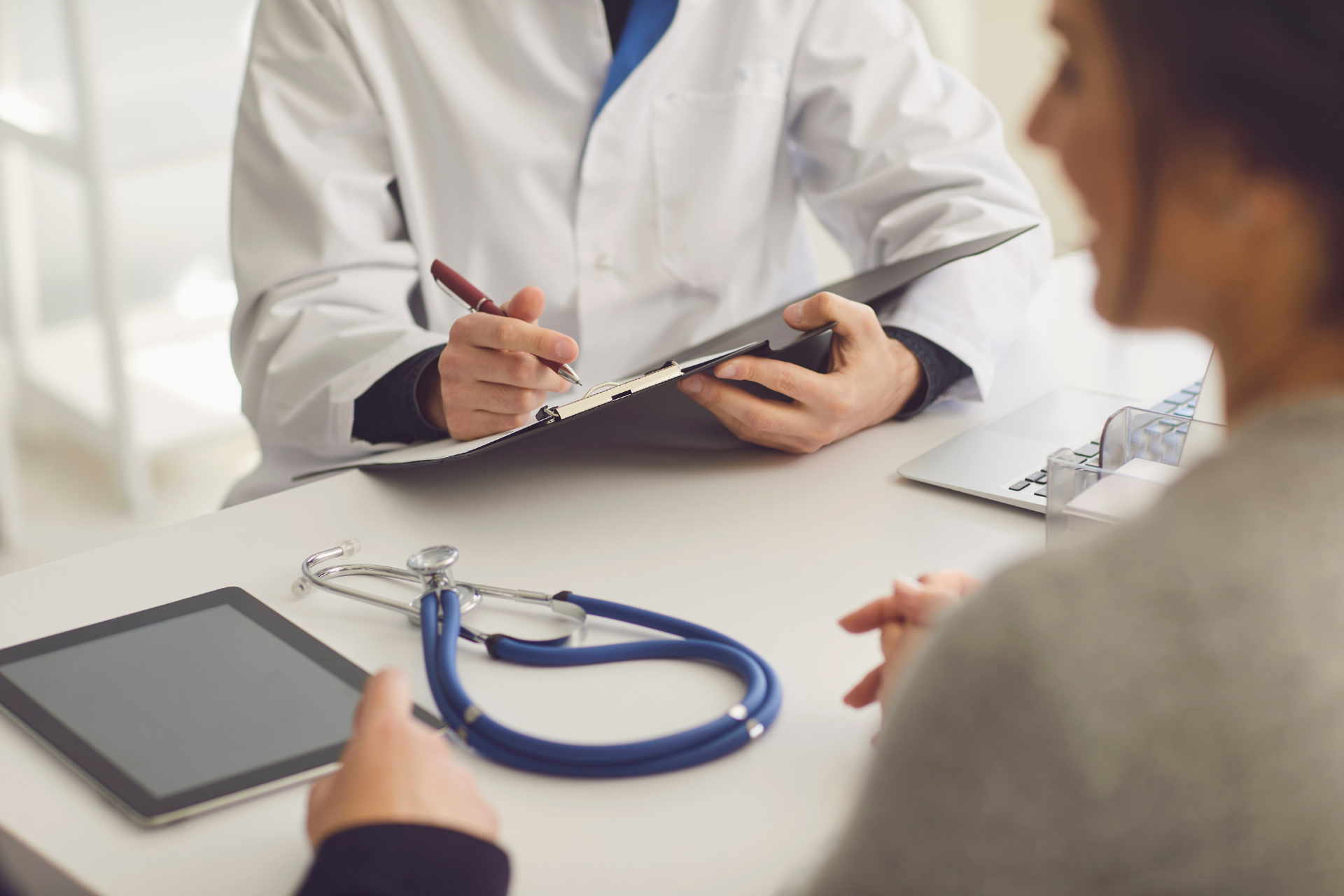
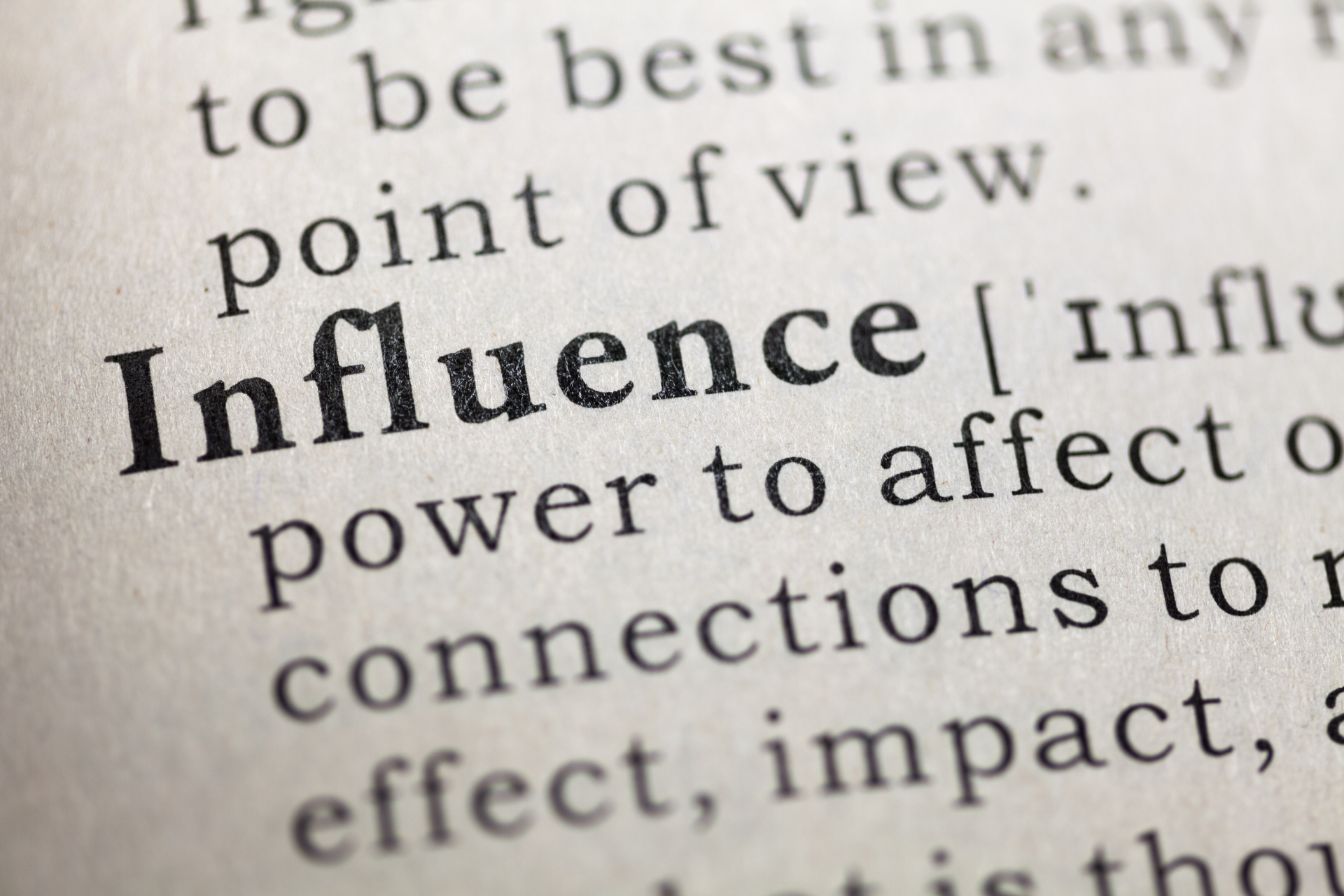
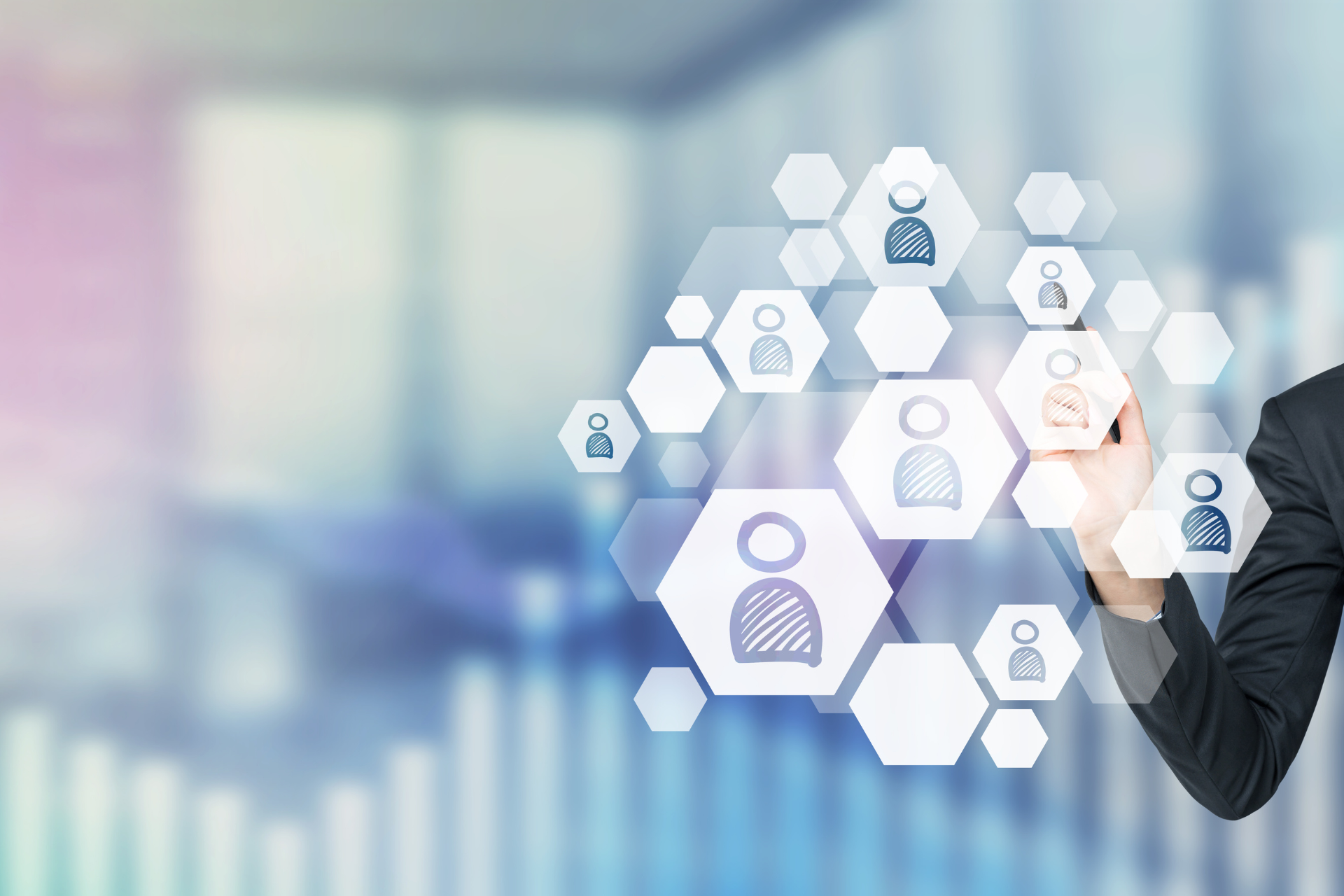